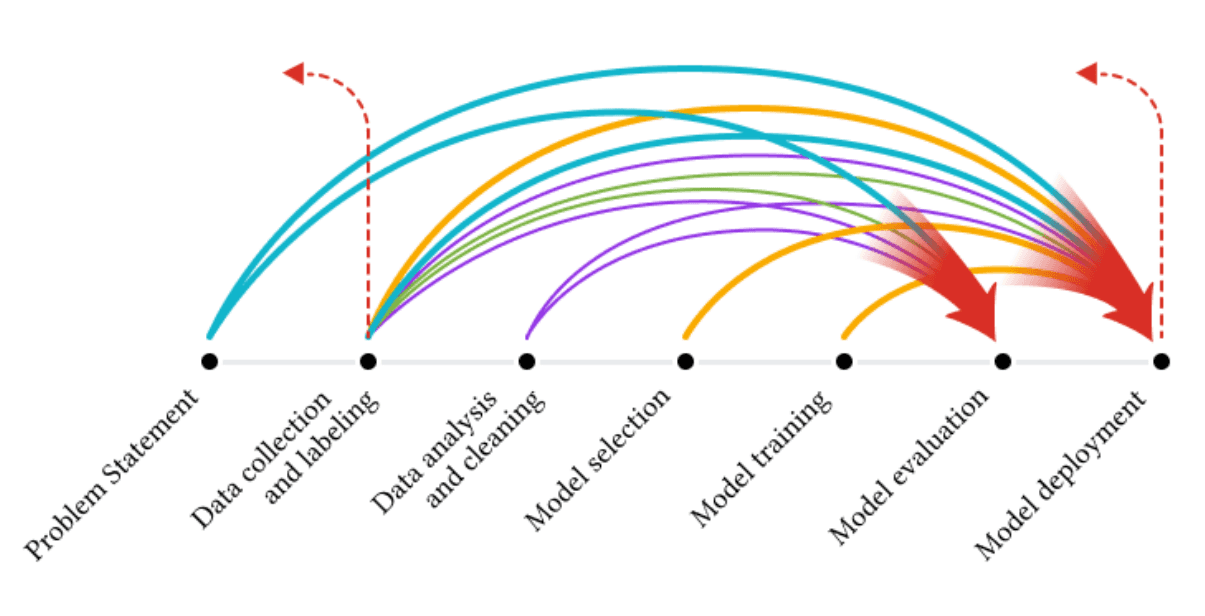
Data cascades and how to prevent them
"Data cascades" are a form of technical debt—implied future costs accrued by design choices made today—that, according to one group of researchers, are especially prevalent in machine learning systems in high-stakes domains such as health and conservation.
Identifying a number of such data cascades, they argue that the most egregious kind—when models" trained on noise-free datasets are deployed in the often-noisy real world"—is nearly ubiquitous in cyberphysical systems that measure real world phenomena. In these settings, after long periods of time (2 to 3 years), models can "drift", as the "brittleness" of physical environments (e.g. a dislodged sensor), hardware failures, changes in the underlying environment that deviate from the model's assumptions, among others. This occurred in more than 90 percent of the cases they examined.
They conclude by highlighting the need for new incentives in machine-learning research—the most relevant for urban tech: a shift from emphasis on goodness-of-fit to goodness-of-data in AI research, and a need for real-world data literacy in AI education. This points towards a future where urban tech may prove to be one of the most problematic domains for AI when it comes to data cascades, and urban tech innovators, procurers, educators, and students need to tool up accordingly. At worst, such data cascades could hamper or undermine the development of the entire field.