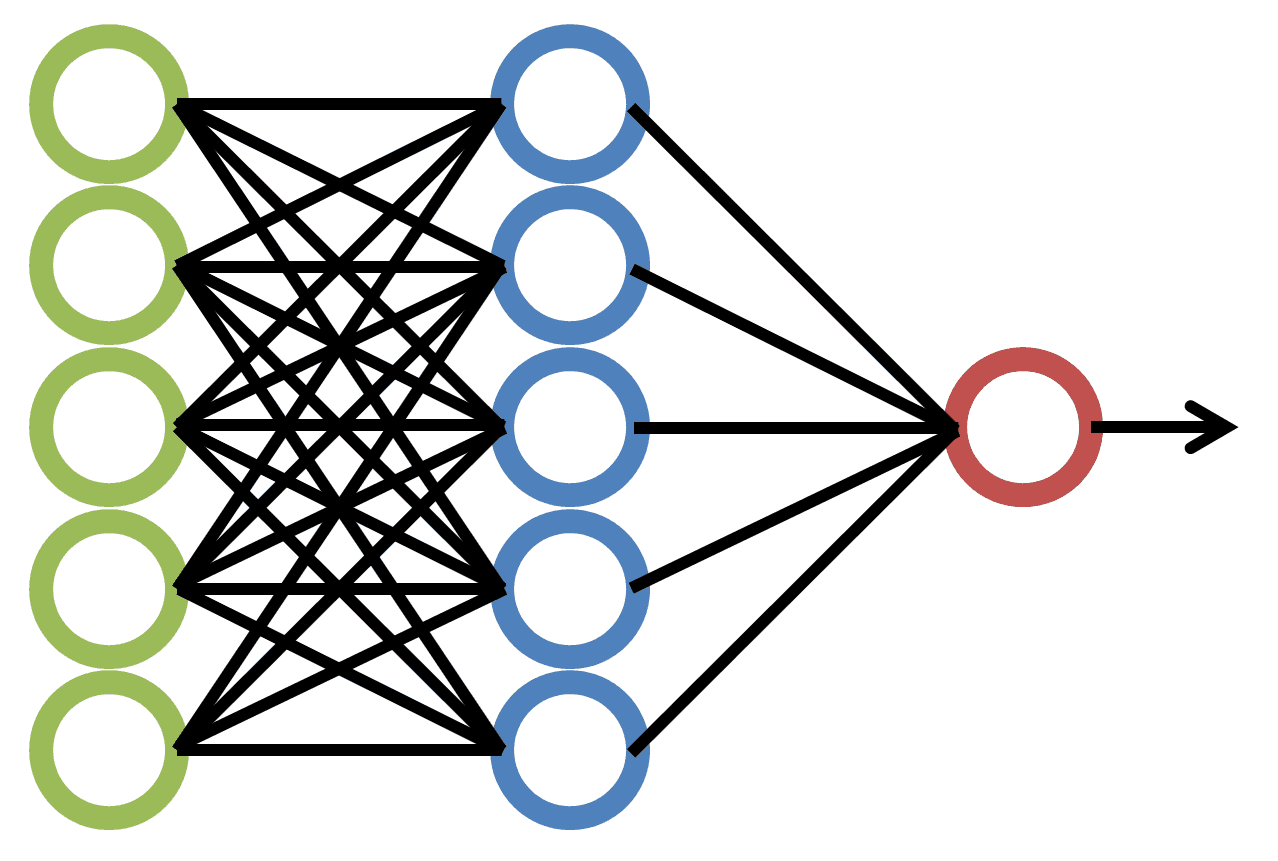
Decentralizing deep learning for carbon emission reduction
Training a large deep-learning model can produce as much CO2 as five cars over their entire lifetime, according to a recent study. What's more, as models become larger and ever more demanding, computational requirements are outstripping hardware improvements. To continue hitting new performance benchmarks, they are consuming exponentially increasing amounts of computing power. "At this rate, the researchers argue, deep nets will survive only if they, and the hardware they run on, become radically more efficient." The source describes multiple promising techniques, which, if they prove successful will preserve this growth trajectory but also produce other benefits—notably moving deep learning from data centers to edge devices, reducing leakage of sensitive data and reducing latency and reaction time, "which is key for interactive driving and augmented/virtual reality applications." This points towards a future in which today's highly centralized cloud infrastructure, especially in urban tech applications, can be dispersed more widely in urban systems—civil infrastructure networks, buildings, and vehicles—allowing for new possibilities for data governance and tie-ins to resilient, renewable power generation.